Algorithmic Bias and Alternative Data: Five Lessons From the DOJ's Meta Settlement
- Richard Pace
- Aug 10, 2022
- 14 min read
Updated: May 6, 2024

Author's Note: Shortly after this article's publication, the Consumer Financial Protection Bureau ("CFPB") issued an interpretive rule stating that technology firms that use sophisticated behavioral targeting techniques to market financial products for financial firms can be held liable by the CFPB or other law enforcers for committing unfair, deceptive, or abusive acts or practices as well as other consumer financial protection violations. Based on this new rule, I have incorporated an additional recommendation for third-party compliance risk oversight within the "Implement Demographic Disparity Testing of Algorithmic Outcomes" section below.
On June 21, 2022, the U.S. Department of Justice ("DOJ") announced a settlement with Meta Platforms ("Meta" aka Facebook) to resolve allegations of discriminatory advertising in violation of the Fair Housing Act ("FH Act"). While recent, the matters at issue in this Settlement have a relatively long and storied public history - including a prior investigation by the U.S. Department of Housing and Urban Development ("HUD") and a 2019 Settlement with the National Fair Housing Alliance ("NFHA"). As such, I will not go into all of the contextual details here. Instead, I simply note that the challenged practices involve Meta's use of its members' demographic information and accumulated alternative data - e.g., personal behaviors, interests, preferences, etc. - together with complex machine learning algorithms to target the distribution of housing-related digital ad content to members of Meta's social media platforms (e.g., Facebook and Instagram).
In addition to the Settlement's direct implications for digitally-enabled target advertising/marketing, what I find notable about this matter is what it tells us about the federal government's broader concerns regarding algorithmic bias, what it demonstrates to us about the tenacity of demographic patterns to infiltrate AI/ML algorithms, what the federal financial regulators' future algorithmic focal points may be under an expanded "fairness" purview, and what types of remedial actions may be required under future algorithmic-focused enforcement orders. However, before turning to these broader lessons, I start with a summary of the algorithmic bias and alternative data issues raised in this matter.
Alleged Explicit and Implicit Biases in Meta's Digital Advertising Platform
The 2019 NFHA Complaint against Facebook primarily focused on disparate treatment under the FH Act caused by Facebook's use of explicit demographic identifiers (or extremely close proxies) to target its members for housing-related ad content. Accordingly, the NFHA Settlement focused on Meta's removal of such explicit demographic attributes from the analytical tools it provides its advertisers to target these audiences.
Since the 2019 NFHA settlement, HUD and the DOJ further investigated Meta's digital advertising platform - including testing the platform for potential audience disparities after Meta's implementation of the settlement's agreed-upon changes. Based on this investigation, the DOJ Complaint found additional disparate treatment issues in ad platform processes not covered by the NFHA Complaint and, more interestingly, identified additional outcome biases that highlight how even "demographically blind" machine learning algorithms can still introduce harmful disparate impact biases into our digitally-enabled financial lives through the effects of alternative data.
In particular, even without access to explicit demographic attributes - nor the intent to target based on such demographics, the government's testing allegedly found evidence that the algorithms still produced biased outputs - indicating that the NFHA settlement was insufficient in de-biasing Meta's housing-related advertising tools. In the next sections, I describe Meta's digital advertising platform and the DOJ's new findings in more detail, and summarize the new remedial actions required of Meta.
Meta's Lookalike Targeting Algorithms
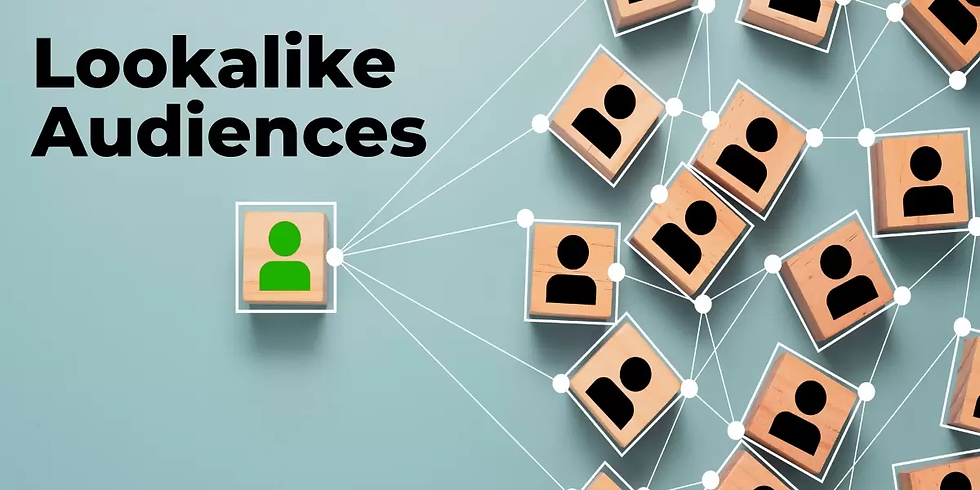
To achieve revenue growth, a company will normally engage in advertising to expand its potential customer base. However, as an alternative to broader-reach advertising within legacy media channels (e.g., magazines, television shows, radio programs, etc.), Meta provides a way to target a company's advertising to highly-prized "lookalike" audiences - that is, members who are either most like the company's existing customer base, or most like the subset of its "best" customers.
To create this lookalike audience, a company provides Meta with an existing customer list to "seed" Meta's machine learning algorithms. Meta then matches this customer list to its massive member database to enrich the customer records with several alternative data attributes derived from the individuals' interactions with Meta platforms and partners. Next, machine learning algorithms are deployed to identify patterns in these attributes that help to differentiate these customers from Meta's general member population. Once these differentiating attribute patterns are identified, Meta then applies them to its broader member database to identify specific individuals who "look like" the customers in the company's original "seed" list. The company can then target its ads to these lookalike individuals - thereby increasing the effectiveness of its digital advertising spend.
The DOJ Complaint refers to this algorithmically-created target audience as the "eligible audience" as it represents the subset of Meta members who are eligible to receive a targeted digital ad according to the lookalike algorithm's results. However, as the next section describes, this eligible audience can be further algorithmically differentiated to improve the efficiency of the advertiser's digital ad spend.
Meta's Personalization Algorithms

Once a company's eligible audience is created by Meta's machine learning algorithms and alternative data, the next step is to deliver ad impressions to these potential customers. However, companies typically have a fixed advertising budget and wish to spend this budget on digital ad impressions that will have the greatest financial return - that is, they wish to further target the ads to those members of their eligible audience that are most likely to find the ad "relevant".[1]
To accomplish this second related business objective, Meta offers additional machine learning algorithms ("personalization algorithms") deployed against its database of member attributes to predict the probability that an eligible audience member would respond to, or engage with, an ad of similar content (i.e., an "ad relevancy probability"). With these probabilities, a company can rank order its eligible audience from highest to lowest ad relevancy probability - thereby spending its fixed advertising budget most efficiently (i.e., on the subset of eligible members with the highest likelihood to engage with the ad).
The DOJ's Alleged Algorithmic Discrimination and Agreed-Upon Remedies
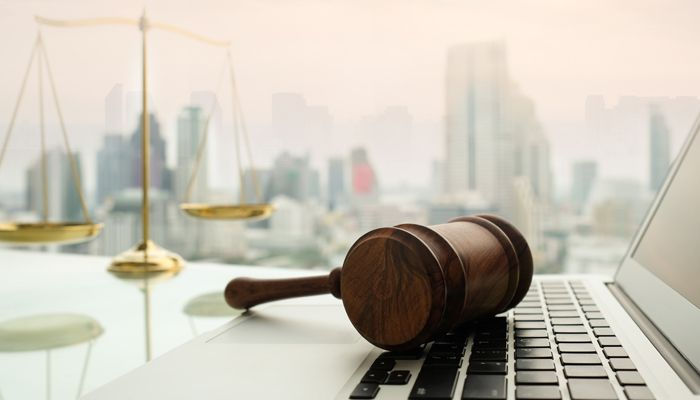
According to the DOJ Complaint, for some time, both of these machine learning algorithms were able to access protected demographic attributes in Meta's member database to create the eligible audiences and estimated ad relevancy probabilities - thereby engaging in allegedly illegal disparate treatment under the FH Act. For example, if a company's "seed" customer list for a lookalike audience was skewed demographically toward Whites, or if males were more likely to engage with ads similar to the company's, then Meta's algorithms could identify and use such demographic patterns to create the company's eligible audience and/or estimated ad relevancy probabilities and, thereby, potentially disadvantage one or more protected class groups through "digital redlining".[2]
However, even more interesting is the allegation that, even after Meta allegedly removed access to demographic attributes from the lookalike machine learning algorithms per the NFHA settlement, the government's testing revealed that the algorithms' outputs were still demographically-biased - creating eligible audiences whose sex and race/ethnicity demographics closely mirrored those of the company's "seed" customer list - an outcome alleged to represent illegal disparate impact under the FH Act. Indeed, per the DOJ's Complaint, Meta's lookalike machine learning algorithms are still incorporating prohibited demographic patterns of "seed" customer lists by leveraging (or creating) indirect proxies for such attributes from Meta's vast trove of alternative data[3], and there appears to be an expectation that Meta's personalization algorithms will suffer the same fate even after access to direct demographic attributes is removed (such removal was not a part of the 2019 NFHA settlement as the personalization algorithms were not in scope).
To resolve these algorithmic bias allegations, Meta agreed to the following remedial actions as part of the DOJ Settlement:
Algorithmic Retirement - as of December 31, 2022, Meta will no longer provide companies with the ability to create lookalike audiences for housing-related advertising using Meta's machine learning algorithms and alternative data.[4] While not explicitly stated, it is likely that this retirement remedy was adopted - rather than algorithmic de-biasing (see below) - because de-biasing would prove extremely difficult with a customer "seed" list whose demographic biases Meta does not control.[5]
Algorithmic De-Biasing - to reduce the disparate impact associated with its personalization algorithms (after removing access to direct demographic attributes), Meta will modify its targeted ad distribution process to minimize disparities between the demographics of a company's eligible audience (i.e., sex and race/ethnicity compositions) versus the demographics of the actual audience to which ad impressions are delivered. However, the exact methodology by which the audience demographic disparities will be eliminated, as well as measured after modification, is still "to be determined" and requires resolution between Meta and the DOJ by December 16, 2022.
Author's Note: In January 2023, Meta released its Variance Reduction System to address this remedial action. My analysis of this algorithmic de-biasing approach can be found in the blog post: Meta's Variance Reduction System: Is This The AI Fair Lending Solution We've Been Waiting For?
The Meta Settlement: Five Lessons For Compliance Officers
Although the DOJ Settlement deals specifically with allegations of digitally-delivered, housing-related advertising discrimination under the FH Act, there are likely broader lessons here with respect to where federal regulators and enforcement agencies are heading with their clear concerns over algorithmic bias in financial services. In what follows, I lay out five potential actions that Compliance Officers should consider in managing similar risks for their institutions.
Ensure Proper Controls Over the Use of Non-Traditional Alternative Data
Using non-traditional alternative data - such as individuals' behaviors, interests, and preferences - in consumer-oriented AI/ML algorithms may significantly increase a financial institution's discrimination risks.
Meta's experience, as well as - to a certain extent - common sense, reveals that alternative data focusing on broad-based consumer behaviors, interests, and preferences may exhibit a more significant degree of variability across demographic dimensions (such as sex, race/ethnicity, and age) than more traditional financial and alternative data - thereby increasing their utility as direct or indirect demographic proxies.[6] This may explain why the algorithmic bias alleged by the government in Meta's lookalike models was barely diminished when access to direct demographic attributes was withheld from the algorithms (i.e., the algorithms easily replicated these direct demographic patterns through their use of this alternative data).
From a risk mitigation perspective, this suggests the importance of compliance policies and controls that limit consumer algorithms' ability to "see" individuals along prohibited dimensions so as to eliminate the use of such dimensions as predictive patterns (even if such patterns are empirically / statistically relevant). However, this requires not only blinding the algorithm to direct measures of these prohibited dimensions, but also any indirect measures that may be - individually or in combination - highly correlated with the prohibited dimensions. This exercise becomes increasingly difficult the more alternative data is available, the greater the complexity of the algorithmic architecture, and the greater the number of predictive data attributes permitted in the algorithm.
Implement Demographic Disparity Testing of Algorithmic Outcomes
With the advice of legal counsel, all applicable consumer algorithms should be empirically evaluated for prohibited demographic biases - with appropriate remedial actions taken in response to such testing.
While there are still many open questions concerning algorithmic bias testing and remediation, Compliance Officers may still need to navigate these turbulent waters to implement appropriate compliance risk management controls today - with the knowledge that: (1) there is currently a high degree of technical and regulatory uncertainty in this area and (2) the definition of "appropriate" controls and remediations will surely evolve over time based on industry and regulator activities. For example, an early step may be to place strong restrictions on the use of alternative data until more clarity emerges on the benefits and risks of algorithmic de-biasing.
To the extent that algorithmic de-biasing is considered as a potential remedial action, Compliance Officers and other key stakeholders should be well-versed in the current varieties of these methodologies - as well as their relative benefits, risks, and limitations. To start, I suggest the information in my prior blog posts "Don't You Forget About Me: De-biasing AI/ML Credit Models While Preserving Explainability" and "Six Unanswered Fair Lending Questions Hindering AI Credit Model Adoption". While these posts are focused on AI/ML credit models, their information is more generally applicable to other AI/ML algorithms used in financial services.
When third-party service providers are engaged to assist your company with the selection of target audiences and/or the distribution of digital ad content, appropriate and reasonable due diligence would be prudent to evaluate whether the provider(s) have sufficient controls and documented evidence that their algorithms and data do not impart illegal demographic biases to your company's advertising / marketing campaigns. Compliance Officers should be integrally involved in this due diligence process, and there should be effective policies that govern required actions for providers who cannot or will not provide such information, or will not cooperate in reasonable disparity testing of their algorithms' outputs.
Finally, for AI credit models, Compliance Officers may also wish to evaluate how the CFPB's recent Circular 2022-03 impacts the use of non-financial alternative data in light of the CFPB's position on the meaning of "specific reasons" for adverse action notifications.
Ensure Proper Focus on Digital Consumer Advertising and Marketing
With respect to digital marketing, prohibiting the use of legally-protected demographic characteristics to target new customers is a necessary, but insufficient, compliance control.
With the CFPB's expanded interpretation and application of the Consumer Financial Protection Act's "unfairness" standard to more general forms of discrimination in the provision of financial products and services, customer acquisition processes are likely to receive increased regulatory scrutiny for potential discriminatory effects. Accordingly, financial institutions should be very careful in how they target or identify potential customers through "lookalike" algorithms or other analytically-based processes.
At a minimum, Compliance Officers should ensure that campaign targeting lists (or "eligible audiences" as defined in the DOJ Settlement) are evaluated for material demographic discrepancies with the corresponding addressable market - that is, the population of individuals meeting basic eligibility requirements for the product or service being expanded.[7] This likely will require that the institution employ demographic proxies to perform such assessments - and consider the risks and limitations of such proxy-based disparities in the interpretation of results.[8]
To the extent that problematic demographic discrepancies are identified, the institution should work with legal counsel and relevant stakeholders to design appropriate modifications to its customer acquisition processes - including exploring methods to remove such biases in its supporting algorithms and analytic tools.
Algorithmically profiling your "best" customers for digital marketing can lead to additional regulatory risks - such as UDAAP.
Outside of financial services, it is quite common to identify "best" customers using measures such as "customer lifetime-value" that estimate the total or net present value of potential revenue and/or profits expected from a customer relationship. With such measures, companies may seek to expand their revenues / profits by profiling and targeting such customers (either existing or new) to maximize return on marketing spend.
Within financial services, however, how one defines "best" customers carries with it some significant legal, regulatory, and reputational pitfalls (in addition to the potential discrimination risks discussed above) - particularly if "best customers" are defined as those that generate the highest fee or interest income. This is because regulators may see the targeting of such individuals as predatory if the associated revenues or profits are not commensurate with a reasonable value exchange. Indeed, targeting current or potential customers who may be more likely to incur late fees or other penalty charges, or who could be slotted into more expensive loan products, may create significant compliance and reputational risks. Accordingly, Compliance Officers should ensure that customer acquisition and customer management processes are subject to appropriate risk and control assessment processes.
Properly Scope Your Algorithmic Bias Compliance Risk Assessments
Ignoring the larger operational processes in which a specific algorithm is embedded may yield an incomplete evaluation of potential discrimination risks.
As described in the DOJ's Meta settlement, the alleged bias of Meta's lookalike algorithms was amplified by their interactions with a second downstream set of algorithms (i.e., the personalization algorithms) that determined the relevancy of a particular ad to the eligible audience members and, therefore, influenced to whom from the eligible audience the ads would actually be delivered. However, the personalization algorithms were not identified for inclusion in the NFHA investigation and settlement - thereby allegedly allowing such algorithms to continue to access prohibited demographic attributes when determining to whom to display a company's digital ad content.
Accordingly, when performing risk assessments for potential algorithmic bias, it is essential to identify the entire end-to-end process in which a particular algorithm is embedded, and to evaluate whether there are additional upstream or downstream algorithms, rule sets, or other analytically-based tools that impact the overall results of the process and, therefore, should be incorporated into the corresponding bias testing (and potential remediation). Additionally, you may also find that a specific algorithm is actually used in multiple consumer-facing processes - in which case your bias testing should be appropriately inclusive of each separate process (for example, a consumer credit scoring algorithm may play a role in credit decisioning, credit pricing, and line amount determinations).
Coda: Who's Wasserstein?
The DOJ's Settlement Agreement contains an interesting, but limited, discussion of Meta's primary remedial action - notably, the creation and implementation of a "Variance Reduction System ("VRS")" to remediate the alleged algorithmic bias in Meta's personalization algorithms.[9] Specifically, according to the Settlement Agreement:
"Meta will develop a system to reduce variances in Ad Impressions between Eligible Audiences and Actual Audiences, which the United States alleges are introduced by Meta’s ad delivery system, for sex and estimated race/ethnicity."
"...Meta will provide an update and discuss with the United States the development, testing, and analysis Meta has done over the last thirty (30) days regarding VRS performance under the Earth Mover’s Distance (“EMD”) or Wasserstein Metric; information regarding how the VRS is performing in terms of reducing variances in Ad Impressions between Eligible Audiences and Actual Audiences for sex and estimated race/ethnicity."
That is, Meta will need to develop a methodology to de-bias the personalization algorithms such that the demographics of the ads actually delivered to Meta members materially align with the demographics of the original eligible audience. For example, if the eligible audience for a company's ad is 11% Black, 14% Hispanic, 5% Asian, and 70% White, then Meta will need to ensure that the actual audience members who receive these ads have the same race/ethnicity (and sex) profiles. However, given that the actual race/ethnicity of Meta members is not definitively known, Meta and the DOJ agreed that the BISG race/ethnicity proxy methodology would be used - at the aggregate level - to estimate these audience distribution demographics.
While there is insufficient information here to understand fully the proposed Variance Reduction System, it appears that Meta is also working on how to measure the difference between the eligible and actual audience demographic distributions under the BISG probabilistic approach, and is proposing the use of the Wasserstein metric (also known as the Earth Mover's Distance) to determine both the magnitude by which the two distributions differ as well as the threshold under which the two distributions would be deemed acceptably equal.
It will be interesting to follow this development as it may suggest a new fair lending metric is on the horizon - with the corresponding need to evaluate and, potentially incorporate, this new metric into existing algorithmic bias compliance risk management programs.
Author's Note: In January 2023, Meta released its Variance Reduction System to address this remedial action. My analysis of this algorithmic de-biasing approach - including the use of a new fair lending metric ("shuffle distance") - can be found in the blog post: Meta's Variance Reduction System: Is This The AI Fair Lending Solution We've Been Waiting For?
* * *
ENDNOTES:
[1] Additionally, Meta's lookalike algorithms produce eligible audiences that have a minimum size equal to 1% of the target location. For some companies, or for some advertising campaigns, this minimum eligible audience size may be excessive relative to the available ad spend budget - thereby requiring a means to further narrow the eligible audience down to fit the companies' desired spending levels.
[2] This is not meant to imply that advertisers wanted to target Meta members for housing-related ads based on protected demographic attributes. However, by not explicitly excluding the consideration of such attributes (which may not have been an advertiser option for the lookalike and personalization algorithms), Meta implicitly permitted the algorithms to consider such attributes and use them if they were deemed sufficiently predictive. Per the government's testing, this lack of explicit exclusion allowed disparate treatment to affect audience targeting. For example, if the company's "seed" customer list underrepresented Blacks, then the lookalike algorithm's target customers likely also underrepresented Blacks - an act of exclusion. Additionally, if Blacks were less likely to engage with similar ad content, then the personalization algorithms likely assigned lower ad relevancy probabilities to Black members of the eligible audience - thereby further excluding Blacks from receiving the targeted digital ads.
[3] The Complaint does not definitively identify the source of the alleged disparate impact. Accordingly, while it could be driven by accessible attributes that are close proxies to prohibited member demographics, it is also possible that the algorithms simply created such proxies through complex combinations of correlated, but otherwise benign, attributes.
[4] Technically, Meta has agreed to implement these remedial actions for U.S. digital ads for housing, employment, and credit.
[5] For example, if a company's customer "seed" list was - at the extreme - all male, then it may be virtually impossible to create a "lookalike" audience for such a demographically-skewed group using the types of alternative data collected by Meta and the associated demographic correlations reflected in such data. Meta may also want to limit its on-going legal liability for contributing to the creation of biased ad audiences relative to applicable anti-discrimination laws.
[6] By "more traditional alternative data", I mean data obtained from a consumer's financial transactions - such as checking account cash flow data - that more directly reflect the consumer's financial needs, resources, and behaviors.
[7] This assumes that these basic eligibility requirements satisfy objective, legitimate business needs and would not be considered themselves as potentially illegal discriminatory attributes.
[8] While this activity will help to identify potential biases in new customer acquisition processes, it is possible that the institution's existing customer base demographics may also be materially misaligned with the relevant addressable market(s) due to legacy processes and potential biases. The decisions to evaluate such potential misalignments and to take corresponding potential corrective action should be discussed with legal counsel and appropriate stakeholders.
[9] I assume the VRS pertains to the personalization algorithms due to Meta's planned retirement of its lookalike algorithms (for housing, employment, and credit-related advertising).
© Pace Analytics Consulting LLC, 2023.